“All of us have been paying attention and seeing the ‘AI high’ for the last several years,” said Ruchita Sinha, MBA, General Partner at AV8 Ventures, during a session on AI Productivity Enhancements for Developing Assets at the 2024 Biotechnology Innovation Organization (BIO) Investor Forum.
We’ve recently seen “large jumps” in funding for AI startups and multiple partnerships, she said. “However, once AI got to the Nobel Prize Committee, and we saw five Nobel prizes awarded in a particular area—and three out of the five awards are at the intersection of AI and biotech—I think we can all agree, it’s time to look past the hype and start thinking about what are the real-world applications.”
Leaders across the biotech industry spoke about how they have incorporated AI into their work and how companies and investors realize a tangible return on investment of AI in their value chain. The conversation covered AI’s potential, pain points of AI, and ways AI is already helping companies work faster and more profitably.
Bio.News interviewed Renee Yao, who heads Ecosystem Business Development in Healthcare Life Sciences at NVIDIA.
The use cases of AI in biotech
AI in the biotech sector has undoubtedly been a game changer, especially when it comes to research, logistics, and planning.
“AI is a really natural extension for us,” said Matt Foehr, President and CEO of OmniAb. “Just given the massive amounts of data that are produced that things like machine learning and big data management can analyze, all those things are very relevant to our business and to serving our partners and discovering faster and getting them to the clinic more quickly.”
AI is allowing biotech companies and their partners to look at larger data sets and find patterns and correlations—and to develop better research models that were impossible (or very slow) to develop before. This is speeding up the rate of discovery.
Alan Joslyn, Ph.D., Chief Executive Officer of Inimmune Inc., discussed his company’s ability to enhance small molecule drug discovery at comparatively lightning speed.
“That’s not always been possible, even in the last five years, to really attribute a value to that whole process,” he said. “The thing that’s really starting to change is the amount of data that we have and the quality of machine learning models. And so we’re building multi-task machine learning models to predict the properties of these small molecules.”
NVIDIA’s pioneering platform
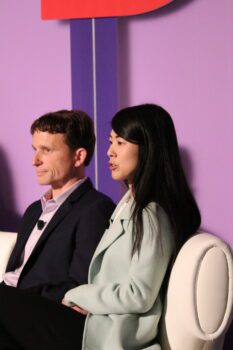
NVIDIA was an early adopter of AI innovation—both within and without the biotech space.
“We built out the BioNeMo platform early. Essentially, it’s a large language model for biology and chemistry,” said Renee Yao, who heads Ecosystem Business Development in Healthcare Life Sciences at NVIDIA. The leading use cases include optimization of target discovery and early drug discovery.
Additionally, AI has proven extremely beneficial when it comes to logistics and planning.
NVIDIA’s Omniverse platform is a digital twin for manufacturing and biomanufacturing, allowing the company to offer services that can envision a building and development plan before it happens. “We can help companies simulate the future vaccine plant or design the next lab in the loop to do virtual factory planning,” Yao explained.
How AI is improving clinical trials
AI is also helping companies plan better clinical trials.
“I think it’s important to kind of compare overall versus new world to see how things really change,” said Michelle Longmire, M.D., CEO & co-founder at Medable. Her company connects patients, researchers, sites, and everyone along the clinical trial pipeline to improve the development, implementation, and monitoring of clinical trials.
“Before, there was no virtual option, and every clinical trial was largely a service-first hard-wired technology solution,” Longmire explained. “What we have done is brought cloud, mobile, and AI solutions to this problem. We created something we call the medical research superhighway.”
“You can think of patients and sites and then infrastructure that can connect them with the scientific and protocol-specific workflows,” she continued. “It’s just been fascinating to see how much value AI can bring to this problem.”
The pain points of AI implementation
AI uptake has been a dynamic process both in the biotech sector and outside of it. Many are worried about learning curves and even losing their job as a result of the new technology.
“We are an organization of chemists, formulators, and immunologists,” said Alan Joslyn, Ph.D., CEO of Inimmune Inc. “We are not an organization of computer programmers or people who can really build out an AI-type system.” Joslyn explained that the company has two chemists trained in AI and machine learning who made several recommendations about how to bring in the software without disrupting the company’s existing workflow.
NVIDIA has been very clear about its intent to incorporate AI into its operating structure without putting jobs at risk.
“We definitely sensed some fear,” Yao said. “I even thought that maybe parts of my job were going to get replaced, and then instantly, when we got the email from our CEO directly to say, None of what you guys are going to get laid off, we just need to prioritize better.”
Incorporating AI into a company structure comes down to shifting company cultures. There will always be employees who are early adopters; the trick is to make sure those who might have less of a technological background, or those with a larger degree of skepticism, eventually buy in and get comfortable with AI as a tool.
ROI for AI
Overall, the panel agreed that AI implementation had significant ROI for companies.
“Our customers really want to get patients into trials,” said Longmirre. “It’s all about speed and cost—and we had a lot of benchmarking data prior to AI, and with AI. So, we focus on being able to show these are the things that matter to drug developers.”
If you can show that the ROI of the AI tracks with the company’s ROI, “it becomes a really straightforward measurement,” Longmirre continued.
On the research side of things, ROI is a bit clearer. AI speeds up research, promotes collaboration and partnerships, and therefore strengthens innovation—a point that each of the panelists made directly.